Articles
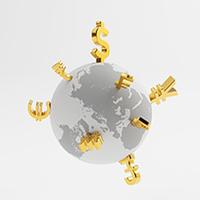
Ubiquity
Volume 2024, Number November (2024), Pages 1-24
Digital Economy: The Digital Economy as a Complex System
Kemal A. Delic, Jeff Johnson
DOI: 10.1145/3679025
The digital economy is a complex system, but orthodox economic theory is unable to handle such complexity. For some decades economists have realized that the conventional theoretical models are not consistent with data on what people and institutions actually do, and a new behavioral economics is emerging. The science of complex systems developed over the last 50 years has developed many new ideas and methods for analyzing the non-linear non-equilibrium dynamics of complex systems. Responding to the failures of orthodox economics, those managing the global economy are willing and able to embrace and lead in this new way of looking at economic systems. Thus, economics is evolving and better able to inform decision making in the public and private sectors.
This offers new ways of sthinking about the digital economy for entrepreneurs and policy makers which in turn will provide many new example of real-world complexity. The digital economy will drive the creation of future wealth and prosperity, co-evolving with the science of complex systems. We postulate that the research in complex systems will enable better understanding of the digital economy, augment existing economic models and improve their prediction powers.
The economy is a highly dynamic system, saturated with uncertainty that makes it impossible to predict using the methods of traditional science. Seen at the global level it involves billions of people, trillions of items, astronomic numbers of transactions and exchanges, and events in a constant flux. From any perspective, it is a complex system.
Complex systems science gives new ways of understanding messy ever-changing systems of systems and their entangled bottom-up, top-down dynamics. It provides ways of investigating their possible future behaviors for decision-making in the private and public sectors. This new science is enabled by computation. This underlies new methods of mathematical, statistical. and computational modeling using huge data sets.
Systems theory emerged in the 1960s in recognition that many of the things being studied across the sciences—including biology, ecology, engineering, economics, business and management—had features common to them all. They all consist of things interacting with each other [1].
At its simplest, a system can be defined to be a set of components connected together in an organized way where the components are affected by being in the system and the behavior of the system is changed if they leave it.
The digital economy is a system with many levels of aggregation from the microlevel of individual people to the macrolevel of governments, international bodies, and multinational corporations. There are many subsystems such as internet marketing, the mortgage and banking systems, food delivery, recommender websites, mobile communications, and so on. At all levels these are complex adaptive systems that interact with their environment, e.g., many start-ups adapt their business as they learn more about their potential customers. At all levels the agents in the digital economy self-organize, e.g., entrepreneurial people come together to form new businesses, companies come together as suppliers and customers, and nations come together as trading partners.
The science of complex systems has developed a rich language to describe the dynamic processes that characterize complex adaptive systems and has developed new theoretical and computational methods that can support the design and management of the digital economy and its many entangled subsystems.
Complex Systems
A key characteristic of complex systems is their ability to reconfigure themselves to create new systems with completely different properties. The emergence of new properties and behaviors can be unexpected, e.g., a flash crash in the financial markets, or they can be the result of planning and design, e.g., the emergence of a new set of customers or followers after the design and launch of a new website.
In general, the behavior of complex socio-economic systems cannot be predicted with certainty and there is no scientific equivalent to the physical science that underlies the highly predictable behavior of machines such as automobiles and aircraft.
Although there is no widely accepted criterion for a system to be "complex" there are characteristics of systems that can make them appear to be complex and unpredictable [2]. These include:
- Many heterogeneous interacting parts
e.g., cities, companies, climate, crowds, political parties, ecosystems - Complicated transition laws
e.g., markets, disease transmission, cascading failure rioting, professional training - Unexpected or unpredictable emergence
e.g., chemical systems, accidents, spontaneous social initiatives, foot and mouth disease - Sensitive dependence on initial conditions
e.g., weather systems, investments traffic jams, forest fires - Path-dependent dynamics
e.g., the evolution of the qwerty keyboard, racial conflicts, first-to-market - Networked hierarchical connectivities
e.g., social networks, ecosystems, the internet, voting systems, postal systems - Interactions of autonomous agents
e.g.,, road traffic, dinner parties housing markets, soccer games, crowd dynamics - Self-organization or collective shifts
e.g., revolutions, fashions, choirs, demonstrations, property rental markets - Non-equilibrium dynamics
e.g., fighter aircraft, share prices, the weather armed conflict, social networking - Combinatorial explosion
e.g., chess, communications systems, data states for a computer program - Adaptivity to changing environments
e.g., biological systems, manufacturing design, retail systems, rebranding - Co-evolving subsystems
e.g., land-use, transportation, computer virus software - Ill-defined boundaries
e.g., genetically modified crops, nations, pollution, terrorism, markets - Multilevel dynamics
e.g., companies, armies, governments, aircraft, Internet, transportation, markets
Any of these properties can make systems unpredictable in the sense that point predictions cannot be made so that the system will be in a particular state at a particular time. However, complex systems are not entirely unpredictable, and the future behavior of the digital economy is not entirely unknowable. It will increasingly be investigated and better understood using the methods of complex systems science which asks, "How do complex systems come into being by co-evolution and self-organization of their parts, and by coevolution and self-organization with their environment, and how do they maintain themselves in their environment without top-down control?"
Sensitivity to Initial Conditions, Dynamical Systems, and Chaos
When you put an origin and destination into your satnav it plots a suggested trajectory in space-time as a wiggly line on a two-dimensional map. Figure 1 shows a trajectory in three-dimensional space through time, specifically the trajectories of the Lorenz system discovered by the meteorologist Edward Lorenz.
In 1961 Lorenz was experimenting with weather prediction. He discovered that making the same calculation with a lower digit precision gave different results. Although the initial difference was very small, repeated calculation amplified it so that the outcomes from the two initial states were completely different and the process appeared to be chaotic. This launched the new mathematical field of dynamical systems. In this theory, there are attractors that bound the system behavior and strange attractors with complicated geometry and non-periodic trajectories. The trajectories of the Lorenz attractor in three-dimensional space are illustrated in Figure 1. An animation can be seen here. It illustrates the idea of a strange attractor, which in this case has two lobes with the system trajectory unpredictably moving from one to the other. Lorenz suggested the possibility that a small change in one part of the system could have a large effect on another part of the system
The idea that small interventions can have significant consequences lies behind the idea of nudge. To make someone do what you want them to, look for interventions that themselves have little cost or require little energy but have the desired result. Thaler and Sunstein give the example of changing the way food is presented to children in a school cafeteria system [3]. In some cases, it was possible to increase or decrease the consumption of food items by 25%. At almost no cost the consumption of some unhealthy food could be replaced by healthier options. Another effective nudge idea researched and implemented by the UK Behavioural Insights Team involved adding the statement "most people pay their tax on time" to tax reminders. Many nudge ideas involve making it easier for people to do what you want them to do, e.g., making it easier to fill in online forms.
Cellular Automata, Self-organization, and the Edge of Chaos
Cellular automata are usually grids of square cells and transition laws that determine the state of a cell at the next "tick of the clock" given the current state of the cell and its neighbors.
Cellular automata can be used to investigate the nonlinear dynamics of spatial systems. For example, more than 50 years ago the American sociologist Thomas Schelling proposed that a mild preference to be located with neighbors in one's own social group would be enough for the formation of ghettos in which the social groups separated. This is illustrated in Figure 2. Figure 2(a) shows two social groups distributed in an area at random. At each tick of the clock, each agent looks at its neighbors. Consider a red agent. If the majority of its neighbors are red it remains where it is. Otherwise, it moves at random to a vacant white cell, which then becomes red. Figure 2(b) shows the system after 10 ticks of the clock where clustering by social group is already beginning. Figure 2(c) shows the system after 100 ticks of the clock, where the ghettos have clearly formed.
Cellular automata enable a wide variety of questions to be answered. For example, suppose a transit company is planning a new e. Building the station involves a considerable investment to be offset by, among other things, buying land at a low price before the station is built and selling the land over time after the station is built. Suppose that land near the station would command the highest price. If this were the only consideration land values would decrease in concentric circles away from the station. However, there may be other complications including, for example, the location of bridges over a winding river. Also, through time there are other effects. As more people move to live close to the station, services and businesses will appear such as cafes, restaurants, newsagents, dry cleaners, travel agents, and possibly even law firms and banks. This process is called agglomeration—local densification of people, infrastructures, and institutions—and it increases land values. The transit company would know this and would hold back the land directly next to the station so that agglomeration could occur after sufficient dwelling plots have been sold further away from the station, increasing land values near the station. A cellular automaton model of this system would enable the developer to investigate land release options for the new station development.
Stephen Wolfram developed "a new kind of science" using cellular automata with black and white cells and a range of transition rules [4]. Wolfram discovered there seem to be four classes of behavior. In Class 1, either all the cells become white, or all the cells become black (stasis). In Class 2, the final state consists of simple structures that may repeat (dynamic equilibrium). In Class 3, the behavior is more complicated with triangles and small structures appearing, but apparently at random. Wolfram wrote: "Class 4 involves a mixture of order and randomness: localized structures are produced which on their own are fairly simple, but these structures move around and interact with each other in very complicated ways" [4]. This intermediate class between regularity and randomness has been called "the edge of chaos."
Networks
In 1934 psychiatrist Dr. Jacob Moreno published a remarkable book in which he used networks in a sociometric study of the New York Training School for Girls, a reformatory school in Hudson, NY. "[I]t has the size of a small village, between 500 and 600 persons; it is a closed community; it has a unisex population; the girls are still in their formative age and remain in Hudson for several years until their training has been completed; they are sent in from every part of New York State by the courts; they are a cross-section of the nationality and social groups of New York. … There are 16 cottages for housing purposes, a chapel, a school, a hospital, an industrial building, a steam laundry, a store, and administrative building, etc., and a farm. The housemother has the function of the parent; all meals are cooked in the house under the direction of a kitchen officer; the girls participate in the household in different functions, as waitresses, kitchen helpers, cooks, launderesses, corridor girls, etc." [5].
Moreno described this community and its rich web of social interactions, which he drew as networks illustrated in Figure 3. The girls are drawn as red circles (nodes in the network). Positive relationships are drawn as red lines (links in the network), and negative relationships are drawn as black lines. Some links are drawn as arrows, showing they are one-way.
There were various problems at the school such as girls absconding and fighting. Moreno, with great insight, attributed the social problems partly to the network structure—the links in the networks and the connectivity between the girls—and used network concepts to design a new social structure less conducive to trouble. Unlike many people managing social systems Moreno did not attribute blame to individuals when their problems arose, e.g., that someone is difficult, disruptive, or bad, but sought network explanations that caused individuals to be difficult, disruptive, or bad.
Network theory has revolutionized our understanding of socio-economic systems. The modern definition is that a network is a set of vertices (nodes) and a set of pairs of nodes called edges (links or arrows). Two vertices are connected if there is a chain of edges between them. The number of edges incident to the vertex is called its degree. There are other useful structural properties of networks and Moreno used many of them.
Three-quarters of a century after Moreno's study, Nicholas Christakis and James Fowler published another remarkable study using networks to demonstrate surprising relationships between people in social systems. For example, your colleague's husband's sister can make you fat, even if you've never met. Also, our friends' friends, and even our friends' friends' friends have an astonishing influence of our behaviors, beliefs, and health.
Christakis writes, "I began by being interested in the simplest social network of all, a pair of people, a dyad. Initially, the dyads I studied were husbands and wives. As a physician caring for terminally ill patients and their families, I noticed the serious toll that a loved one's death had on a spouse. And I became interested in how illness in one person might cause illness in another. For it seemed to me that if people are interconnected their health must also be interconnected. … To know who we are, we must understand how we are connected" [6].
Christakis and Fowler discovered a rich data set for their work—the Framingham Heart Study that began in 1948 and continues to run in the present day. Initially, two-thirds of the adults in Framlingham, MA, signed up to be examined by doctors every two years, and since then many of their children have also signed up. These data included detailed information about the friends, relatives, coworkers, and neighbors of each participant. A network was painstakingly mapped out of more than 50,000 links by focusing on a group of 5,124 people within a larger network of 12,067 people. Researchers used the data to investigate changes in obesity over time and found it was socially contagious. Many other things were investigated, including smoking cessation. These too followed paths in the social networks.
Many networks have long-tailed distributions, as illustrated in Figure 4. Most of the nodes for this network have low degrees with one or two edges, but a few have high degrees with as many as nine. These networks have the small world property that all the nodes are connected by relatively short chains of intermediate nodes, e.g., most people on earth are six handshakes away from everyone else. The number of contacts people have on social media is a long-tailed distribution. Some people have very high numbers of contacts while most people have many fewer.
Long-tailed distributions are common in real systems; Figure 5 shows the distribution of the numbers of billionaires by country has a long-tailed distribution. A few countries have a lot of billionaires, and a lot of countries have few billionaires.
Networks play a central role in spreading viruses, where one person infects another, and infection is transmitted along chains of links. In the case of COVID-19, many governments attempted to stop the spread of the infection by disconnecting the social network by imposing "lock downs" with most people restricted to their homes.
Network theory has concepts and tools for analyzing the spread of information in social systems, including theories of percolation as things are transmitted through populations, e.g., infection, values and beliefs, radicalization, conspiracies, fake news, and gossip. Cascades occur when exponentially increasing numbers of agents are affected over time. These occur, for example, when a person with a large Twitter (X) following retweets a message, and others with large followings also retweet it.
Rethinking the Financial Network
"On 15 September 2008, Lehman Brothers filed for Chapter 11 bankruptcy in a New York courtroom in the United States. Panic ensued. Uncertainty about its causes and contagious consequences brought many financial markets and institutions to a standstill. … Banks hoarded liquidity for fear of lending to infected banks, causing gridlock in term money markets,"—remarked, Andy Haldane, formerly of the Bank of England, in a speech to the Financial Study association Amsterdam (FSA) in the midst of the global financial crisis (GFC) [7].
In a 2010 speech, Jean-Claude Trichet, then President of the European Central Bank, said, "When the [2008] crisis came, the serious limitations of existing economic and financial models immediately became apparent. … As a policy-maker during the crisis, I found the available models of limited help. In fact, I would go further: in the face of the crisis, we felt abandoned by conventional tools" [8].
Since then, it has been realized that the global financial system is a complex system and must be modeled and analyzed using the methods of complex systems science, including network science.
The financial network is robust yet fragile, meaning it can tolerate random component failures, such as isolated business failures, but is vulnerable to the failure of highly connected nodes such as the collapse of Lehman Brothers. Haldane illustrates this by the increasing connectivity of the international banking network (see Figure 6). The nodes are countries, and the links represent cross-border stocks of external assets and liabilities. "It is clear that the scale and interconnectivity of the international financial network has increased significantly of the past two decade. Nodes have ballooned, increasing roughly 14-fold. And links have become both fatter and more frequent. The network has become markedly more dense and complex" [7].
The failure of Lehmann Brothers illustrates a new economic perspective. In networks, the impact of the failure or success of a node depends on how that node is connected.
Networks and Behavioral Economics
Network theory is having a revolutionary effect on many areas of economic thinking. For example, orthodox economics theorizes that rational decision-makers assess all the options and choose the best. This theory assumes the decision-making agent has perfect information about the choices available and evaluates them all. Nobel Prize winner Herbert Simon challenged the empirical basis of this. For many choices such as the purchase of a car or a telephone there are thousands of options and evaluating them all would give the agent "cognitive overload"—for most decisions people don't have the time or inclination to collect and assimilate the information available on thousands of options. Simon proposed instead bounded rationality where the choices of "rational" agents are bounded by their ability to collect and process information. Whereas some people look to recommender websites for guidance, for many the source of information is their social networks such as asking their friends or tech-savvy children.
Over the last few decades behavioral economics has emerged as an approach that takes into account psychological, cognitive, emotional, cultural, and social factors involved in decisions made by individuals, companies, institutions, and policymakers. Behavioral economics looks for empirical evidence about how agents—people, firms, and governments—behave in practice.
Paul Ormerod writes, "At the micro level, however, economics does give powerful insights into how the world operates. Indeed, it provides us with what is probably the only general law in the whole of the social sciences: individuals react to changes in incentives. In other words, if the set of incentives which an individual faces alter, the agent (a generic term for the individual in economics) may alter his or her behaviour" [9]. He gives the example of a driver approaching a speed camera. Without the camera, there is a low incentive to keep to the speed limit and a driver may exceed it. However, there is a strong incentive to obey the limit when there is a camera, and the driver may change their behavior and slow down. When the camera is passed the incentives change and the driver may speed up.
In many cases this new approach implicitly or explicitly uses networks. For example, consider a government policy aimed at helping long-term unemployed people back into work. Some policies have been tried that target the individual, for example requiring people to accept possibly unsuitable jobs or lose benefits. Taking a network view, the social network of unemployed young people includes other unemployed young people, and they are disconnected from the networks of people who know where there are opportunities for work. This suggests that new network-oriented policies could be applied to this problem, including the links and connectivity as well as providing incentives for the nodes.
A similar argument can be applied to youngsters who drift into crime. If caught the punishment is served by the individual, but crime is a network phenomenon, the social network of a young criminal is likely to include other young criminals. When a young person has served a custodial sentence, they need a support network and if this is not provided recidivism is very likely.
An example of policy success is the way the UK handles speeding penalties. For minor transgressions, drivers are allowed to attend a course on speeding. The course costs about €80, about the same as a fine, but the incentive of avoiding penalty points attracts most drivers. Importantly, the courses form small networks of about 10 people. During the course, an instructor shows videos explaining how each extra mile per hour greatly increases stopping distances. For most people in the network, this is surprising new information. The instructor then requires individuals to speak to the group on various safety aspects of driving, the speeding penalties of fines, higher insurance premiums, or even loss of licenses. Generally, the group forms a collective view that speeding is undesirable, and most individuals aim to change their behavior. In terms of achieving the policy goal, it is a success—participation in a speed awareness course cuts reoffending rates by as much as 23% after six months compared with those drivers who were offered a course but turned it down.
Perhaps one of the greatest policy failures in modern times is the "war on drugs" declared 50 years ago when Richard Nixon, the 37th president of the United States, declared drug abuse to be public enemy number one. Drug abuse is a network phenomenon. If you take drugs those in your social networks are also likely to take drugs. Drugs are distributed locally by networks of suppliers to their networks of customers. Well-intentioned campaigns, such as "Just Say No," target the nodes in the networks but do not address the network structures such as groups of friends. Furthermore, the drug problem is a system of systems. The consumer networks interact with the supply networks in complicated ways. "Just Say No" is a failed policy that targets the nodes in networks but not the link structures.
Network science is giving new insights into the constraints on human behavior. Our social networks clearly play a considerable role in what we do as individuals. For example, many people subscribe to LinkedIn, the social media platform used by professionals to network with others for mutual benefit. It is not uncommon for people to have hundreds of LinkedIn contacts. The idea behind LinkedIn is that connected members can form communities (highly connected clusters in network terminology) that add value to their members. Sometimes new contacts are made when people request a connection, and sometimes LinkedIn suggests people to connect with, and it is very easy to accumulate many contacts. One might think the more contacts the better. In fact, there are considerations similar to bounded rationality—can one really interact with many hundreds or thousands of contacts? In the 1990s the British anthropologist Robin Dunbar suggested humans could have about 150 meaningful relationships, now known as "Dunbar's number." However, other researchers have questioned this, saying that people making the effort could have a much greater number.
Social Networks and the Internet
The internet is an essential part of the digital economy. It is a huge infrastructure network that supports an astronomical number of social and economic networks. Billions of people use the internet daily and it is an essential part of work and leisure. Many things have been improved by the internet, but some disturbing new things are emerging.
Social media is having a great impact on our children in ways that we don't understand. Growing up was always difficult, but these new kinds of interactions are making it more difficult for some. Children can enter downward spirals of depression as they are attracted to content that matches their low moods. Jonathan Haidt warns the great rewiring of children's brains by social media is causing an epidemic of mental illness [10].
Adults too are impacted in new ways by the internet. It has become a channel for anonymous hate and threats of violence. Our legislators are particularly affected. Most, especially women, experience extreme vitriol for their political positions and stated views, and many regularly receive terrifying threats. Without doubt, this is an existential threat to our democracy. So too is the abuse of social media to collect data on individuals and target them with political messages. So too are fake news and politically motivated lies. So too are conspiracy theories. These problems can be added to economically motivated cybercrime and politically motivated cyberespionage.
Some think the anonymity that is fundamental to the design of the internet is a severe flaw that needs correction. Others disagree arguing that anonymity encourages innovation. Be that as it may, there are many aspects of the internet, and societies adapting to it, that require new policies to prevent the harm that is being done. It is certain that network theory will play a major role in this.
Models in Economics as Theory Of Economy
Describing the real-world functioning economy is a huge, long-standing, challenging problem with a long history of studies of economic structure and performances. An example of this early effort is economist Bill Phillip's MONIAC (Monetary National Income Analogue Computer) to simulate the British macroeconomy, which he created in 1949: "By controlling taps and valves and observing the flows of liquid, trainee economists learned how to model the economy using mathematical equations" [11].
Figure 7 gives a conceptual view of the economy. It depicts the process of creating models driving policies and shaping regulations based on predictions, forecasts, and assessments. In modern times, large computational machinery runs big models and large simulations of much larger scale and scope —but serving again the same purposes—to understand the underlying mechanics and behavior of the real economy.
Economics is the study of markets, aiming to understand fundamental mechanisms, predict market movements, and interpret different behaviors. It is a group of studies embodying the theory of the real-world, functioning economy. However, orthodox economics is not well adapted to the highly dynamic nature of the digital economy. For many years the eminent "complexity economist" Brian Arthur developed new ways of describing the economy as an organic and evolving complex system [12, 13, 14].
For example, the theory of diminishing returns of orthodox economics does not capture the market behavior of the digital economy. In the 1980s Arthur challenged the orthodox view with his theory of increasing returns [15].
The following passage from Arthur's personal website provides some background on his work on increasing returns: "In standard economic theory, markets show diminishing returns. There are only so many good hydroelectric sites in Norway and after these are exploited hydro energy runs into diminishing returns—it becomes more costly. So hydro and petrolium [sic] energy share the market. But some economic markets show increasing returns. If one company or product or technology gets advantage it gains further advantage—there are increasing returns or positive feedbacks. So when several such companies compete, one that by chance or clever strategy gets ahead may gain further advantage and can go on to dominate or lock in the market…. Arthur's original 1983 paper on this was turned down by 4 top journals over a period of 6 years. Increasing returns seemed rare and esoteric—not quite 'economics.' Finally the paper was published in the Economic Journal. (Currently its citations are over 12,000.) The theory wasn't much noticed at first, but became prominent when it was realized that high-tech markets operated under increasing returns: If a technology has a network of users and gets ahead, this enlarges its network and increases its advantage, so it can go on to lock much of the market. By the 1990s Silicon Valley had embraced increasing returns, and since then the theory has been standard for tech markets" [16].
It is difficult to formally represent evolving, dynamic systems as a real-world, functioning economy. The reason is that the economy—in general—is a messy and unpredictable system. Scientists are attracted to elegant formulas and equations for which they have made unrealistic assumptions—so that the models are nice and elegant, but rarely fit the reality of a functioning economy [17, 18]. Consequently, the results of running those models are sometimes not reliable, typically inaccurate, and quite often wrong. Knowing that they are feeding policies, legislations, and regulations, one may wonder what research on complex systems can contribute to improving it [19, 20].
Digital Economy Framed
The digital economy is observed as a coincidental rise of the internet, the growth of the web, a massive spread of mobile telephony, and big data. This has created a dependable infrastructure for omnipresent connectivity, always-on availability, and maximal mobility. The boom and the bust of the web at the turn of the millennium triggered the first e-commerce applications as a prototype of digital services later evolving into something called the "new economy" [21]. Two and half decades later, society is profoundly transformed, so that our private and professional lives are heavily dependent on the digital world, connectivity, and service economy.
For illustration, some rough estimates of the size and structure of the digital economy are given in Figure 8. Some recent estimates gauge the world economy as a $100-plus trillion system. Using a simple Pareto principle approach, one can assume that the contemporary digital economy amounts to $20 trillion. Of those, probably 80% represent various digital services and 20% are digital products.
Modeling the Digital Economy as a Complex System
The digital economy is a system of systems of systems. Its intersecting and highly entangled subsystems include those on the left as shown in Figure 9. All of these subsystems have some or most of the complexity characteristics as shown on the right in Figure 9. Complex systems science is already used in many areas of the digital economy, e.g., e-marketing already makes extensive use of network theory, and the UK government uses nudges on its websites.
We believe in the future methods of complex systems science will be applied across the digital economy. For example, health will increasingly move toward personalized medicine using the methods of complex systems science. An e-education curriculum will adapt to individual learning styles and path-dependent educational experiences, e.g., missing lessons through illness.
Conventional economics models the world through equations such as those representing supply and demand to determine prices at equilibrium. But during the 2008 crash Trichet "found the available models of little help [and] abandoned by conventional tools"[8]. It is now clear that modeling economic systems, including the digital economy, requires a "non-equilibrium social science" [22] and computer simulation.
In Making Sense of Chaos (Allen Lane, 2024), J. Doyne Farmer offers the following tenets [23]:
- "The simulations used in complexity economics are called agent-based models. As the name implies, the individual building blocks of these models—such as households, firms or governments—make decisions; they have agency. Algorithms describing how each agent makes decisions are programmed into the computer. As the agents gather information and make their decisions, their actions change the state of the economy, which in turn generates new information, causing the agents to make new decisions, and so on."
- "In agent-based models, economic phenomena emerge from the bottom up, just as they do in the real world."
- "There are many advantages to using simulations rather than mathematical equations, but one of the most important is to model the diversity of the actors in the world—what economists call heterogeneity."
- "Computers, in contrast, easily keep track of diverse agents, making it possible to do this as realistically as needed.''
The micro-foundations of the systems in the digital economy can be explored using agent-based modeling. For example, consider modeling the road traffic of a city with a million people. This can be done using hypotheses as to how people choose their origin, destination, and route. Some trips involve other people, for example, a mother may drop of their children at nursery, drop off the father at his workplace, and end the trip at her own workplace. Thus, data is required not just of the individual agents but also of their family structure. Of course, no city has such data for a million people. However, more aggregate data will be available including census data. This can be used to create a synthetic micropopulation with a million agents. Also, there will be national data on family structures, and this can be used to form relations for synthetic agents.
Whatever aggregate data is used, the synthetic micropopulation has the same statistical structure as the data.1 This approach is becoming increasingly used to simulate the behaviors of social systems, e.g., a synthetic micropopulation for the entire United States was created to study the impact of alternative policies for transportation restrictions during the COVID-189 pandemic.
Complex systems are usually modeled in the context of policy and decision-making to get the "best" outcome. There is a large literature on optimization, usually involving mathematical models. Behavioral economics with its focus on evidence over theory shows that for many problems there is no optimum. Herbert Simon challenged the theoretical notion of their being optimum solutions to problems or decisions with the best possible outcomes. Instead, he proposed the idea of satisficing where, for practical reasons such as running out of time, decisions are made that are acceptable on all dimensions—good enough overall—but not necessarily perfect on any of them.
Brigid Rosewell, a prominent economist with extensive business experience writes, "Also, although innovation is a pillar of economic policy, its necessary dynamics are incompatible with equilibrium theories. Equilibrium also suggests that 'do nothing' is a policy option for no change, rather than drifting into the unknown. However, 'doing something' has the additionality problem, namely showing the benefits over doing nothing or something else. All investment impacts are on the balance of probabilities. Risk free investment and risk free polices are not possible in a complex world. What matters is to have a strong story, backed up by strong evidence on the main elements of the story. Then take a bet" [24].
Modeling complex systems takes many forms. For example, Dirk Helbing has investigated the way people move in restricted places using video recordings and computer simulations based on mathematical models. It is remarkable that people can walk down crowded streets without bumping into each other, and this can be understood through computer simulations. Applications of this mathematical computational theory include the design of emergency exits in buildings and stadiums. In emergencies, panic can inhibit people from leaving buildings. Pushing and shoving can cause jams as two or more individuals get stuck in a doorway or a restricted corridor. Helbing and Johansson have shown that redesign can make emergency evacuation more effective [25].
Possible Future Developments
Looking into the next few decades, we will see the rise of big digital companies, dominating the digital economy—as is already the case today; with a few trillion-dollar companies ("Magnificent 7") thriving in and dominating the digital economy with tens of trillion dollars in market value. We envision an inversed value pyramid based on a few chip-producing companies feeding the construction of trillions of edge sensors, devices, and systems running a huge variety of applications embodying digital services. This gives an instance of a multilayer, multiscale system-of-systems model of digital economy infrastructure.
A very simple model of digital economy layers indicates key driving factors and major players (see Figure 10) that can be used to better understand the forces driving this growing field. The fundamental ingredients of the digital economy are chips produced by a limited number of companies able to deliver volume and quality at acceptable pricing points. The entire chip market is roughly divided into chips running in gigantic data centers and chips driving edge devices. They represent a fundamental block for application markets divided into two big digital services camps (walled garden versus open marketplace) as innovative revenue-sharing platforms for digital corporations and application authors. Today, there are an estimated 5 million applications in total.
We are aware of the large impact of cyber currency/digital money, AI advancement, and the emergence of new AI devices, which are discussed within this symposium, and we will not give details here. However, some segments of the economy will be changed beyond recognition based on these technological impacts.
The digital economy by speed, scale, and scope is very different from the classical, non-digital economy. Acceleration goes by 1000s, scale by 100s, and scope by 10s. Therefore, it is highly likely that digital economy models cannot directly reuse classical economic models—they might be eventually modified. We believe an argument that some complex systems models—macro, micro, and mesoeconomic models—will be the best choice for digital economy modeling. As digital and non-digital economies are interdependent and interacting, we can presume that a meta model, combining the two, would be the most realistic outcome.
Conclusions
The digital economy is a complex system, but orthodox economic theory is unable to handle such complexity. For some decades economists have realized that the conventional theoretical models are not consistent with data on what people and institutions actually do, and a new behavioral economics is emerging. The science of complex systems developed over the last 50 years has developed many new ideas and methods for analyzing the non-linear non-equilibrium dynamics of complex systems. It seems that those managing the global economy are willing and able to embrace and lead in this new way of looking at economic systems. The digital economy will drive the creation of future wealth and prosperity, co-evolving with the science of complex systems.
References
[1] von Bertalanffy, L. General Systems Theory. George Braziller, New York, 1969.
[2] San Miguel, M. et al. Challenges in complex systems science. The European Physical Journal Special Topics 214 (2012), 245–271.
[3] Thaler, R. H. and Sunstein, C. R. Nudge: i=Improving decisions about health, wealth and happiness. Penguin Books, London, 2008.
[4] Wolfram, S. A New Kind of Science. Wolfram Research Europe Ltd, 2002, 235.
[5] Moreno, J. L. Who Shall Survive: A New Approach to the Problem of Human Relations. Nervous and Mental Disease Publishing Co., Washington D.C, 1934, 69–70.
[6] Christakis, N. and Fowler, J. Connected: The Amazing Power of Social Networks and How They Shape our Lives. Harper Press, London, 2010.
[7] Haldane, A. "Rethinking the financial network." Speech given at the Financial Student Association, Amsterdam. Bank of England. April 28, 2009
[8] Trichet, J.-C. "Reflections on the nature of monetary policy non-standard measures and finance theory." Opening address at the ECB Central Banking Conference at Steigenberger Frankfurter Hof Hotel. Frankfurt, Germany. November 18, 2010.
[9] Ormerod, P. Economics. In Johnson, J. et al (Eds.) Non-Equilibrium Social Science and Policy: Introduction and essays on new and changing paradigms in socio-economic thinking. Springer, Cham, 2017, 21; https://doi.org/10.1007/978-3-319-42424-8_2
[10] Haidt, J. The Anxious Generation: How the great rewiring of childhood is causing an epidemic on mental illness. Penguin Press, New York, 2024.
[11] Science Museum. How does the economy work? December 4, 2018.
[12] Arthur, W. B. Positive feedbacks in the economy. Scientific American 262, 2 (1990).
[13] Arthur, W. B. Complexity and the economy. Science 284, 5411 (1999).
[14] Arthur, W. B. Foundations of complexity economics. Nature Reviews Physics 3 (2021).
[15] Arthur, W. B. On competing technologies and historical small events: The dynamics of choice under increasing returns. IIASA Working Paper. WP-83-090. IIASA, Laxenburg, Austria, 1983.
[16] Arthur, W. B. Increasing returns. W. Brian Arthur. Santa Fe Institute, 2022.
[17] Farmer, J. D. and Foley, D. The Economy needs agent-based modelling. Nature 460, 7256 (2009).
[18] Farmer, D. Economics needs to treat the economy as a complex system. Working paper. University of Oxford, 2012.
[19] Battiston, S. et al. Complexity theory and financial regulation. Science 351, 6275 (2016).
[20] Axtell, R. L. and Doyne Farmer, D. Agent-based modeling in economics and finance : Past, present and future. INET Oxford Working Paper No. 2022-10. University of Oxford, 2022.
[21] Wilson, D. S. Evolving the new economy: Tim O'Reilly and David Sloan Wilson. David Sloan Wilson Online Archive. February 13, 2022.
[22] Johnson, J. et al. (Eds.) Non-Equilibrium Social Science and Policy: Introduction and essays on new and changing paradigms in socio-economic thinking. Springer Open, 2017. ISSN 1860-0832 ISSN 1860-0840 (electronic).
[23] Farmer, J. D. Making Sense Of Chaos: A Better Economics for a Better World. Allen Lane, Dublin, 2024.
[24] Rosewell, B. Complexity science and the art of policy making. In Johnson, J. et al. (Eds.) Non-Equilibrium Social Science and Policy : Introduction and essays on new and changing paradigms in socio-economic thinking. Springer, Cham, 2017.
[25] Helbing, D. and Johansson, A. Pedestrian, crowd and evacuation dynamics. In Meyers, R. (Eds.) Encyclopedia of Complexity and Systems Science. Springer, New York, 2009.
Authors
Kemal A. Delic is a senior visiting research fellow at the Center for Complexity and Design at the Open University. He is the co-founder of AI-Inc, Ltd. and a lecturer at the University of Grenoble and the University of Sarajevo. He is an advisor and expert evaluator to the European Commission. He previously held positions as a senior enterprise architect and senior technologist and scientist at Hewlett-Packard.
Jeffrey Johnson is professor of complexity science and design at The Open University, CEO of Vision Scientific Ltd, co-founder of AI-Inc, and a past president of the Complex Systems Society. His research interest is developing hypernetwork theory for the science of complex multilevel multidimensional systems of systems of systems. This includes mathematical and computational modeling applied to robotics, vision, AI, and the design and management of public and private sector organizations.
Footnotes
1. https://biocomplexity.virginia.edu/sites/default/files/staff/madhavtut2.pdf
Figures
Figure 1. The Lorenz Attractor (Source: Wikipedia).
Figure 2. A cellular automata simulation supports Schelling's theory of social separation.
Figure 3. Jacob Moreno's network analysis of inmates' social networks in the Hudson community.
Figure 4. Most real networks have a long-tailed distribution and a small-world property.
Figure 5. The number of billionaires by country in 2024 has a long-tailed distribution.
Figure 6. Networks of cross-border stocks between countries from 1985 to 2005 [7].
Figure 7. Conceptual view of the digital economy
Figure 8. Digital economy indicative estimates approximately $20 trillion.
Figure 9. All aspects of the digital economy are complex.
Figure 10. Digital economy pyramid: Key layers and players.
This work is licensed under a Creative Commons Attribution International 4.0 License. Copyright 2024 is held by owner/author.
The Digital Library is published by the Association for Computing Machinery. Copyright © 2024 ACM, Inc.
COMMENTS