Articles
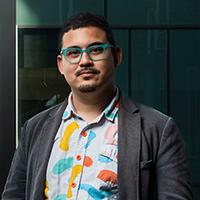
Ubiquity
Volume 2023, Number November (2023), Pages 1-6
Innovation Leaders: A Conversation with Ken Holstein: Fostering human-AI complementarity
Bushra Anjum
DOI: 10.1145/3632842
Ubiquity's senior editor Dr. Bushra Anjum chats with Ken Holstein, an assistant professor at Carnegie Mellon University, where he leads the Co-Augmentation, Learning, & AI (CoALA) Lab. We discuss how, amidst all of the current AI hype, human ability and expertise remain underappreciated. Designing for complementarity in AI-augmented tooling ensures that domain-specific worker-facing AI systems are designed to bring out the best of human ability rather than simply attempting to, many a time incorrectly, automate them away.
Ken Holstein is currently an Assistant Professor in the Human-Computer Interaction Institute at Carnegie Mellon University, where he leads the Co-Augmentation, Learning, & AI (CoALA) Lab: https://www.thecoalalab.com/. CoALA focuses on supporting effective forms of AI-augmented work in real-world contexts, and on scaffolding more responsible AI development practices in industry and the public sector. Throughout their work, Holstein and his team draw on approaches from human-computer interaction, AI, design, psychology, statistics, and the learning sciences, among other areas. Their work has been covered by outlets such as PBS, Wired, Forbes, and The Boston Globe.
What is your big concern about the future of computing to which you are dedicating yourself?
AI systems are increasingly used to augment human work in complex social and creative contexts, such as social work, education, design, and healthcare. Often, these technologies are introduced with the promise of overcoming human limitations and biases. But AI judgments in such settings are themselves likely to be imperfect and biased, even if in different ways than humans.
I am interested in designing for complementarity in AI-augmented work. For me, this means ensuring that worker-facing AI systems are designed to bring out the best of human ability, rather than simply automating activities that humans do best, and that they enjoy or find personally meaningful.
Across a range of real-world workplace settings we've studied, we find that worker-facing AI tools often hurt more than they help, because their designs are based on impoverished understandings of human work and expertise in a given domain. And the frontline workers who are asked to use these systems tend to see their designs as "missed opportunities" to genuinely enhance and complement their abilities. As part of our research, we go out into workplaces that are beginning to experiment with some form of AI augmentation (e.g., decision support tools). We study how these technologies are designed, how they are integrated into organizations, and how human workers actually use them day-to-day. Through our research in social services, K-12 education, and healthcare settings, we have found that worker-facing AI tools are often designed with a focus on substituting rather than complementing human workers' cognitive capabilities, even in cases where humans have comparative advantages over AI. In the context of decision support tools, this can manifest through AI tool designs that present workers with conclusions (e.g., predictions or recommendations), but without empowering human workers' own sensemaking of available evidence, including complementary knowledge they may have as human experts. Relatedly, we have found that the designs of AI-based decision support tools in these settings are often fundamentally misaligned with the actual decision-making tasks and objectives of trained human workers.
Without an accurate understanding of human workers' actual strengths and limitations, worker-facing AI tools are often not designed to address workers' most pressing challenges, and inaccurate assumptions about the nature of workers' tasks and expertise get baked into the metrics that are used to evaluate AI systems' performance. This leads to misleadingly rosy pictures of these systems' usefulness, which do not reflect what actually happens when a system is deployed and used in practice.
How did you first become interested in designing for complementarity in AI-augmented work?
I have long been fascinated with human learning and expertise. My first introduction to research was in the area of computational cognitive science, where I focused on empirically and theoretically studying gaps between human and machine cognition. In particular, I was interested in understanding how humans are often able to learn and infer so much about the world from so little information (relative to the comparatively vast quantities of data required by state-of-the-art machine learning systems).
Amidst all of the current AI hype, I feel that human ability and expertise does not receive nearly the appreciation it deserves. For example, research shows that 11-month-old infants are able to draw rich, accurate inferences about others' internal beliefs, goals, and intentions based upon very short, low-resolution videos of their behavior. This is one of many cognitive capabilities, observed even in human babies, that currently defies automation. Adult human experts exhibit countless more remarkable capabilities, which AI systems are nowhere close to replicating.
During my Ph.D., I moved into the area of human-computer interaction (HCI), where my research focused on developing AI-based technologies to support human teaching and learning and evaluating their use in practice. As part of this research, I worked with K-12 teachers to understand their day-to-day experiences working with AI-based tutoring software in their classrooms, and to co-design and prototype new possibilities for AI to support their teaching. Overall, teachers perceived that existing AI software had been designed with a vision of automating their students' instruction while leaving them largely out of the loop. They saw many opportunities to redesign AI-based tutoring software with the aim of augmenting and amplifying their own abilities as teachers—beyond simply automating instructional interactions with students—in order to empower them to do more of the work that they do best. For example, during class sessions where students work with AI tutoring software, the teacher typically walks around the room and peeks at students' screens to get a sense of what they are working on, and whether they might benefit from the teacher's help. Teachers envisioned future AI systems that could actively assist them during class, helping them assess which of their students would most benefit from their help at a given moment, and with what challenges.
My interests in designing for human-AI complementarity in real-world work settings grew out of these research experiences. These interests have also been reinforced over time, as I've observed similar challenges in other contexts, such as social services and healthcare.
Please tell us more about the CoALA Lab and the projects you are leading to foster human-AI complementarity.
We are leading a set of efforts to overcome these challenges, targeting various points across the AI development lifecycle—from the earliest problem formulation stages, to the design of evaluation approaches and metrics, to the development of worker-AI interfaces. I'll give a few brief examples below.
In one strand of our research, we are exploring ways to improve how AI development teams design new AI systems, beginning with how they generate and select among ideas for AI innovations in the first place. Our goal is to help teams in both public and private sector contexts identify AI project directions that are more likely to produce real value for workers and those they serve, and that carry less risk of harm in deployment. For example, in current research led by PhD researcher Anna Kawakami, we are collaborating with public sector AI developers, agency leadership, and community advocates to co-design a new deliberation process and toolkit, aimed at helping public sector agencies make better informed decisions about which AI projects to pursue.
In a second strand of our research, we are working to improve how AI systems are evaluated in practice. For example, in our prior research we have found that state-of-the-art approaches for comparing human versus AI performance can artificially stack the cards in favor of the AI system: making AI performance look better than it actually is, while making human performance look worse by comparison. To address this, in research led by Ph.D. researcher Luke Guerdan, we are working to develop new evaluation methods [1, 2] that can better capture human strengths that are currently overlooked, and thereby provide fairer assessments of human versus AI performance.
In addition, in a set of ongoing projects we are exploring approaches to AI evaluation that engage end-users and impacted groups in the process of evaluating AI systems. We believe it is critical that the design of evaluations is not left solely to AI developers, as these evaluations need to reflect the knowledge, values, and desires of those who will actually use and be affected by AI systems. For instance, in research led by Ph.D. researcher Tzu-Sheng Kuo, we are developing new methods and tools to support community-driven evaluations of AI systems. Today, various AI-based content moderation tools are deployed in online communities on platforms like Wikipedia and Reddit. We are exploring ways to support members of online communities in collaboratively creating AI evaluation datasets that reflect their collective goals and values for content moderation. Such datasets can then be used by community members and AI developers to understand whether a proposed AI system is truly "fit for use" in their context. In another project led by Ph.D. researcher Wesley Deng, we are exploring the development of new tools and online platforms to engage end-users of AI systems in testing and auditing these systems for behaviors that may be harmful to other users.
Finally, in a third strand of our research, we are exploring the design of new kinds of worker-AI interfaces, to foster human-AI complementarity. As an example of a specific project: we are studying the impacts of decision support and training interfaces that help people learn about and reflect upon "model unobservables:" the information that they have access to as humans, but which an AI model does not. In many of the real-world settings we study, frontline workers have access to a lot of decision-relevant information that AI systems cannot access or cannot interpret effectively. In K-12 education, for instance, teachers may have rich knowledge of their students' personalities, emotional states, and home life. Similarly, healthcare workers may have knowledge of situational or cultural factors that impact what information a patient is comfortable providing about their medical history, versus which details they may omit. However, we've found that in practice, frontline workers will sometimes attribute knowledge to AI systems that these systems do not actually have (or, in some cases, cannot possibly have). In experimental studies, we've found that helping people better understand how their own perceptual abilities complement those of an AI model can actually enable them to better calibrate their reliance on AI when it's time to make decisions. Beyond decision support systems, we are also exploring how best to design co-creative tools to foster human-AI complementarity. For example, in a project led by Ph.D. researcher Frederic Gmeiner, we are currently developing new interfaces to help designers and illustrators get more value out of AI-based design tools.
If you are interested in learning more about our research and its implications, or if you are interested in exploring opportunities for collaboration with our group at CMU, please reach out via our lab contact form (https://www.thecoalalab.com/#get-involved) or get in touch with me directly at kjholsteATandrewDOTcmuDOTedu.
References
[1] Guerdan, L., Coston, A., Wu, Z. S., and Holstein, K. Ground(less)truth: A causal framework for proxy labels in human-algorithm decision-making. In Proceedings of the 2023 ACM Conference on Fairness, Accountability, and Transparency (FAccT '23). ACM, New York, 2023, 688–704. https://doi.org/10.1145/3593013.3594036.
[2] Guerdan, L., Coston, A., Holstein, K., and Wu, Z. S. Counterfactual prediction under outcome measurement error. In Proceedings of the 2023 ACM Conference on Fairness, Accountability, and Transparency (FAccT '23). ACM, New York, 2023,1584–1598. https://doi.org/10.1145/3593013.3594101.
Author
Bushra Anjum, Ph.D., is a health IT data specialist currently working as the Senior Analytics Manager at the San Francisco based health tech firm Doximity. Aimed at creating HIPAA secure tools for clinicians, she leads a team of analysts, scientists, and engineers working on product and client-facing analytics. Formerly a Fulbright scholar from Pakistan, Dr. Anjum served in academia (both in Pakistan and the USA) for many years before joining the tech industry. A keen enthusiast of promoting diversity in the STEM fields, her volunteer activities, among others, involve being a senior editor for ACM Ubiquity and the Standing Committee's Chair for ACM-W global leadership. She can be contacted via the contact page bushraanjum.info/contact or via Twitter @DrBushraAnjum.
Copyright 2023 held by Owner/Author
The Digital Library is published by the Association for Computing Machinery. Copyright © 2023 ACM, Inc.
COMMENTS