Articles
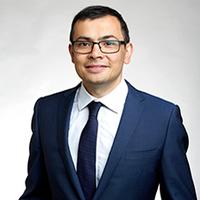
Ubiquity
Volume 2023, Number October (2023), Pages 1-5
Interview with Demis Hassabis, CEO and Co-Founder of DeepMind by Ruth Fulterer of the Neue Zürcher Zeitung
Martin Antony Walker
DOI: 10.1145/3627105
The large-language-model-based chatbot ChatGPT was released on November 30, 2022. Presented here is a translation of an interview held in February 2023 between Ruth Fulterer, of the Swiss-German newspaper Neue Zürcher Zeitung (NZZ), and Demis Hassabis, CEO and co-founder of Google DeepMind. Ubiquity senior editor Martin Walker omitted most comments by the interviewer and details of the interviewer's questions. Although the interview was conducted more than eight months ago, it is full of prescient statements for today's AI. We expect the wisdom expressed by Demis Hassabis in this interview to remain relevant for some time to come.
The large-language-model-based chatbot ChatGPT was released on November 30, 2022. Ruth Fulterer, of the Swiss-German newspaper Neue Zürcher Zeitung (NZZ), interviewed Demis Hassabis, CEO and co-founder of Google DeepMind, about ChatGPT in February 2023.
Although the interview was conducted more than eight months ago, it is full of prescient statements for today's AI. We expect the wisdom expressed by Demis Hassabis in this interview to remain relevant for some time to come.
The following is a translation from German by Ubiquity senior editor Martin Walker, omitting most comments by the interviewer and details of the interviewer's questions. This interview was originally published on February 6, 2023. Permission to republish the interview has been granted by the NZZ.
Ruth Fulterer (RF): Your reaction to ChatGPT
Demis Hassabis (DH): I was surprised at the reactions of users. A couple of questions remain open: it hallucinates occasionally, giving incorrect answers, which is typical for AI language models. It can be impressive, but not reliably so. How many errors can be tolerated when giving medical advice? Learning systems cannot be fully tested—error rate zero is very difficult.
RF: Will DeepMind take the same approach?
DH: As a researcher, I was disappointed at how inelegant the solution to the AI language model was simply was data and brute-force computing power. Yet that brings the best result, so we do that too.
[Bear in mind that the interview took place eight months ago. Hassabis hinted the competing chatbot from Google will take advantage of the information Google has collected from the Internet. Google has built a powerful database containing sorted knowledge that could complement the AI language model. Also, the Google DeepMind system will be able to quote sources. A beta version will come soon. Bard, a smaller version of Google's LaMDA (language model for dialogue applications) is in testing and will be released in the coming weeks.]
RF: What is your proudest achievement?
DH: AlphaFold is already accelerating research on diseases and medicines. That we were able to respond to a challenge that had been around for decades shows what potential there is in AI. The current most promising DeepMind research, jointly with the Swiss École Polytechnique Fédéral de Lausanne (EPFL), is how to control plasma in a fusion reaction (there are publications in Science and Nature). We have helped a couple of leading mathematicians with conjectures. We also work in quantum chemistry. Scientific progress can be accelerated in all domains. It helps that I like to read and want to know about all fields of science. It may sound silly, but one of my hobbies is quantum physics. Many of my friends are quantum physicists and talk about their research. I met the protein folding problem in my first year at university—a friend was possessed by the problem and bored everyone about it. I listened carefully and concluded that the problem was very interesting and could be a candidate for AI.
RF: What makes a research question suitable for AI?
DH: What our algorithms are good at is to model the underlying dynamics in data. The algorithms build a model, which can be used to find the best solution from a vast number of options. In the case of the game Go, the data come from real and simulated games. The solution sought is the smartest move. There are so many possible different sequences of moves that one cannot evaluate all of them. The AI systematically seeks promising paths with its model and then recommends a move. That is the basic principle of AlphaGo. AlphaFold works similarly, only in this case the AI is looking for protein structures.
In every case, we seek advice from experts. With them we clarify whether we have understood the basic problem correctly, We consider together which approaches might work. We usually employ an expert for one or two years in order to precisely define the problem the AI should solve.
RF: DeepMind's mission includes developing a truly intelligent machine; is a system like ChatGPT on the right path?
DH: It is interesting that the approach has changed. When we began to test AI on video games and Go, we were oriented by the brain. Not concretely, for that, we don't understand the brain well enough, but brain research gave inspiration for the construction of those early algorithms. Now the engineers have taken over: bigger models, more data. That moved us away from biological brains. We don't learn a language by reading the whole Internet. This scaling has advanced us, but we observe that important things are lacking—long-term memory, planning, [and] acting in the real world. In my opinion, we need the building blocks for genuine intelligence. The large models are one component, perhaps necessary, but certainly not sufficient for general AI.
RF: This thing you call general AI, how will we recognize it when it's in front of us?
DH: Perhaps in creativity. At the moment we only see two of three steps of creativity. The first is synthesis—put together something new from what has been seen. Generative systems like ChatGPT and Dall-e do that. The second stage is to extrapolate. AlphaGo is a good example. The legendary move 37 in the second game had never been made by a human player. All the experts believed the AI had made a mistake. Then it turned out to have been a move of genius. In 3,000 years, no one had considered it. Now, all Go players use it. That is obviously creativity through extrapolation. But could our system have invented Go or chess? Certainly not. And this third kind of creativity would be a property by which we would recognize genuine AI.
RF: And how is it with consciousness?
DH: We could spend an entire evening discussing that already because we lack a definition of consciousness. Why do I assume you are conscious? Because you behave in a way that I expect a conscious being to behave. But a very good computer program could one day simulate such behavior. Therefore, I believe my conclusion about you works for us because we are similarly constructed. Our brains are made of the same stuff. But computers are made of silicon. It would therefore be difficult for us to recognize a conscious machine.
Author
Martin Antony Walker's (Ph.D. 1969, supervisor Sir Roger Penrose) career has spanned research in mathematical physics, and high-performance computing product research, development, and marketing with several leading high-tech firms in Canada, the USA, and Europe. He has evaluated EC research, advised UNESCO programs, and operated a scientific computing consultancy prior to retirement. His current interest is in applications of AI to science.
2023 Copyright held by the Owner/Author.
The Digital Library is published by the Association for Computing Machinery. Copyright © 2023 ACM, Inc.
COMMENTS