Articles
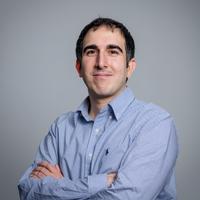
Ubiquity
Volume 2020, Number August (2020), Pages 1-5
Innovation Leaders: A conversation with Gürkan Solmaz: situation classification in the internet of things (IoT)
Bushra Anjum
DOI: 10.1145/3417343
In this interview, Ubiquity's senior editor Dr. Bushra Anjum chats with Dr. Gürkan Solmaz, a senior researcher at NEC Laboratories Europe, Germany, about his work focused on building new situation classification frameworks for smart cities (IoT). They then discuss the three major design aspects for such systems, namely, certainty, efficiency, and privacy.
Gürkan Solmaz is a senior researcher at NEC Laboratories Europe, Germany. His research interests include AI/ML, mobile computing, and cloud-edge systems aspects of IoT with a particular focus on crowd mobility in smart cities as well as autonomous vehicles/drones in IoT applications. He received his B.S. degree in computer engineering from Middle East Technical University (METU) in Turkey and his M.S. and Ph.D. degrees in computer science from the University of Central Florida (UCF) in the USA. He co-authored more than 35 papers, he was co-recipient of two best paper awards and the UCF Computer Science Ph.D. Student of the Year First Runner-up award. He serves in the technical program and organizing committees of IEEE conferences, and he has been a member of IEEE, ComSoc, ACM, SIGMOBILE, and the ACM Future of Computing Academy (FCA).
What is your big concern about the future of computing to which you are dedicating yourself?
The internet of things (IoT) has been expanding through an increased number of IoT devices and the vast deployment of sensors. Simultaneously, there have been significant advancements in artificial intelligence (AI), in particular machine learning (ML), due to the increased availability of data and computation capabilities. We consider that IoT enhanced with AI would enrich our lives in the future. Currently, my research focuses on combining IoT and ML for what we call "situation classification." Situation classification targets recognizing real-world events and generating insights for an accurate understanding of the environment's dynamics. I have a particular interest in the situation classification for the future smart cities and smart mobility IoT applications, where we generate insights for relevant events happening in these domains. For instance, we may detect situations, such as accidents, emergencies, and traffic congestions in smart cities, in real-time for the responsiveness of the city to such incidents. Moreover, IoT data captured from various sensors may enable more efficient crowd management [1] and improved public safety. Lastly, autonomous systems such as autonomous driving vehicles [2], robots, and unmanned aerial vehicles would certainly benefit from situation classification by accurately understanding their environments and making decisions and planning based on their enhanced perspectives of the environments.
As situation classification would benefit society in such obvious ways, the question becomes how to design and build IoT and ML systems, which would be widely applicable and useful for situation classification. Hence, these days we dedicate our research work to answer this question. The existing situation classification systems are mostly tested in specific and controlled (well-calibrated) setups. Applying them into other areas or regions causes a lack of accuracy without any guarantee for successful operation. Moreover, the scalability of these systems to larger regions remains a challenge, as computation and communication complexities arise due to large data volumes. Lastly, situation classification systems should not violate people's privacy by collecting, storing, and processing personal data from devices such as cameras or smartphones.
How did you become interested in the domain of IoT?
During my Ph.D., I had the chance to learn about complex mobile computing and communication problems involving the collaboration of agents such as robots, people, and autonomous vehicles. In the early phase of my research, I noticed solutions to these problems could promote public safety. For instance, in urban areas such as theme parks in Orlando, FL (the city where my university was located), real-time perception of the agents' activities and intelligent collaboration of these agents can help us handle problems of safety, security, and even mitigate the effects of possible natural or man-made disasters.
After my Ph.D., I have had the chance to work in various research projects related to smart cities and smart mobility. In proof-of-concepts projects in collaboration with NEC New Zealand and Australia, we built IoT systems [2] to support crowd management by understanding crowd mobility behaviors in urban areas such as shopping malls and train stations. In recent years, I worked in the AUTOPILOT EU project (No. 731993) to enhance automated driving with the help of IoT. Throughout this project, I periodically visited the Technical University of Eindhoven (TU/e) in the Netherlands, where we conducted field tests with our custom-built autonomous vehicle on the campus. Our work in this project aimed to support the public safety of automated driving through IoT, using sensors from the vehicle as well as the roadside and pedestrians [2].
Working in projects, collaborating with people, and attending various science and tech events shaped my perspective and helped me learn more about the IoT domain problems in terms of aspects such as mobile (edge) computing systems, networking, and machine learning.
Please share more about your recent work and contributions on enabling situation classification for smart cities?
The recently started LOCUS EU project (No.871249) aims to enhance context-awareness related to people and things. In this project, we consider building new situation classification frameworks for smart cities to understand crowd mobility patterns and generate valuable insights from the detected or predicted real-world situations using IoT and ML. For building such frameworks, we consider three major design aspects: certainty, efficiency, and privacy. Let me briefly describe why we focus on these aspects.
Certainty. We consider many existing systems as "siloed" solutions that (are assumed to) operate accurately in specific places and times, during controlled or "wild" experiments. However, there is no guarantee or trust [2] that these solutions can generalize to other environments or adapt to the dynamic changes. The design of systems without certainty may cause much harm, especially for autonomous systems, such as the self-driving car that crashed in 2018. The knowledge-driven approach, by extracting and feeding the domain knowledge, or the data-driven approach, by validation in different temporal/spatial domains, may provide more certainty.
Efficiency. If you check the "top IoT projects" on Google, you may find most of them are in reality, the "toy IoT projects." One of the main reasons is the lack of efficiency of these IoT and ML systems for large-scale applications. Edge/fog and serverless computing approaches can make the IoT systems scalable, by optimizing development, deployment, and resource usage.
Privacy. Although most corporations, governments, and even citizens of certain countries do not seem to care much about it, as IoT and ML would gather vast amounts of data, privacy would be a crucial aspect. In recent years, people in Europe and the U.S. have become more aware of privacy issues. Processing private data through "explainable AI" as opposed to "black-box AI" can be a way forward. The future of IoT (and the future of computing in general) depends highly on creating trustable, explainable, and privacy-aware technologies by design.
I believe future technologies that satisfy these three aspects would be widely applicable. Therefore, I currently lead a research initiative in this direction. If you want to learn more about our approaches or explore possible collaboration opportunities, please feel free to contact me by email at gsolmazATgmailDOTcom.
References
[1] Solmaz, G., Wu, F.-J., Cirillo, F., Kovacs, E., Santana, J. R., Sanchez, L., Sotres, P., and Munoz, L. Toward understanding crowd mobility in smart cities through the internet of things. IEEE Communications Magazine 57, 4 (2019), 40–46.
[2] Solmaz, G., Berz, E. L., Farahani, M. D., Aytac, S., Cheng, B., Fürst, J., and Ouden, J. d. Learn from IoT: Pedestrian detection and intention prediction for autonomous driving. In Proceedings of ACM MobiCom'19, Workshop on Emerging Smart Technologies and Infrastructures for Smart Mobility And Sustainability. ACM, New York, 2019.
Author
Bushra Anjum is a software technical lead at Amazon in San Luis Obispo, CA. She has expertise in Agile Software Development for large scale distributed services with special emphasis on scalability and fault tolerance. Originally a Fulbright scholar from Pakistan, Dr. Anjum has international teaching and mentoring experience and has served in academia for over five years before joining the industry. In 2016, she has been selected as an inaugural member of the ACM Future of Computing Academy, a new initiative created by ACM to support and foster the next generation of computing professionals. Dr. Anjum is a keen enthusiast of promoting diversity in the STEM fields and is a mentor and a regular speaker for such. She received her Ph.D. in computer science at the North Carolina State University (NCSU) in 2012 for her doctoral thesis on Bandwidth Allocation under End-to-End Percentile Delay Bounds. She can be found on Twitter @DrBushraAnjum
©2020 ACM $15.00
Permission to make digital or hard copies of all or part of this work for personal or classroom use is granted without fee provided that copies are not made or distributed for profit or commercial advantage and that copies bear this notice and the full citation on the first page. To copy otherwise, to republish, to post on servers or to redistribute to lists, requires prior specific permission and/or a fee.
The Digital Library is published by the Association for Computing Machinery. Copyright © 2020 ACM, Inc.
COMMENTS