Articles
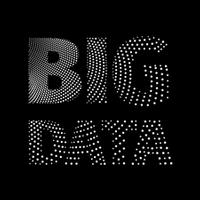
Ubiquity
Volume 2018, Number January (2018), Pages 1-7
Ubiquity Symposium: Big data: big data for social science research
Mark Birkin
DOI: 10.1145/3158339
Academic studies exploiting novel data sources are scarce. Typically, data is generated by commercial businesses or government organizations with no mandate and little motivation to share their assets with academic partners—partial exceptions include social messaging data and some sources of open data. The mobilization of citizen sensors at a massive scale has allowed for the development of impressive infrastructures. However, data availability is driving applications—problems are prioritized because data is available rather than because they are inherently important or interesting. The U.K. is addressing this through investments by the Economic and Social Research Council in its Big Data Network. A group of Administrative Data Research Centres are tasked with improving access to data sets in central government, while a group of Business and Local Government Centres are tasked with improving access to commercial and regional sources. This initiative is described. It is illustrated by examples from health care, transport, and infrastructure. In all of these cases, the integration of data is a key consideration. For social science problems relevant to policy or academic studies, it is unlikely all the answers will be found in a single novel data source, but rather a combination of sources is required. Through such synthesis great leaps are possible by exploiting models that have been constructed and refined over extended periods of time e.g., microsimulation, spatial interaction models, agents, discrete choice, and input-output models. Although interesting and valuable new methods are appearing, any suggestion that a new box of magic tricks labeled "Big Data Analytics" that sits easily on top of massive new datasets can radically and instantly transform our long-term understanding of society is naïve and dangerous. Furthermore, the privacy and confidentiality of personal data is a great concern to both the individuals concerned and the data owners.
Many bold claims have been made about the importance of big data in research [1]. It is 10 years since the need for a new kind of sociology has been urged, moving away from traditional, small-scale randomized samples and focus groups, and exploiting market research and commercial surveys for academic investigation [2]. At around the same time, ideas of "crowd-sourcing" data through citizen networks started to appear [3]. In science more broadly, it has been argued a "data deluge" in fields as diverse as bioinformatics, astrophysics, environmental monitoring, and engineering has created the platform for a new paradigm of data-intensive scientific investigation [4].
In the intervening period, the range and extent of new sources of big data have become almost bewildering. In our everyday lives, we may be leaving a digital trace from the time we wake up (interaction over social media at the breakfast table perhaps) to the time we go to bed (a film downloaded for late evening viewing). In the meantime, our behavior may be monitored through retail activity (supermarket loyalty cards), smart tickets (bus or train), vehicle sensors on roads or in car parks, financial transactions, mobile phone routing, or visits to a gym or a favorite restaurant, to name only the most obvious. Even through the night, smart devices in the household may continue to monitor energy consumption or even the quality of our sleep.
Despite this abundance, academic studies that exploit novel data sources are pitifully scarce. The primary reason for this is simply that data is typically generated by commercial businesses, or in some cases government organizations, and these data owners have no mandate and little motivation for sharing their assets with academic partners. Two partial exceptions to this observation could be made for social messaging data, in particular Twitter, and for some sources of open data. In the case of the latter, the mobilization of citizen sensors at massive scale has allowed the development of impressive infrastructures such as Open Street Map [5]. In the case of the former, the ability to sample freely and easily from the "Twitter firehose" has led to studies ranging from studies of criminal behavior [6], political voting, financial markets [7], population health [8], and even the advancement of the seasons. A strong case can now be made, especially in the case of Twitter, that the widespread availability of these data are driving the applications. In other words, the problems are being prioritized because they can be addressed with social messaging data, rather than because they are inherently important or interesting. Consider, by contrast, what might be achieved with the kinds of data from mobile telephone calls and traces. Major companies (like Telefonica and Vodafone) are capturing millions of customer telephone calls every day. Even when devices are not in active use they are usually pinged several times each hour to determine their status (on/ off) and location. Such data are a potential treasure trove for social scientists with a concern for movement patterns in space and time. This has been identified for more than 50 years as a research priority (e.g. Hagerstrand) and is a field in which developments in theory and application have been restricted by the lack of available data.
The major problem for social scientists with a care for big data can thus be restated in these simple terms: How to gain access to the data which are the most useful and interesting, rather than those which are simply cheap or readily available? In the U.K., this challenge is currently being addressed through significant forward-looking investments from the Economic and Social Research Council in its Big Data Network. A group of Administrative Data Research Centres have been tasked with improving access to data sets in central government (e.g. pensions, tax, transport) while a group of Business and Local Government Centres have been tasked with improving access to commercial and regional sources.
The Consumer Data Research Centre (CDRC) forms a substantial part of this network. CDRC is directed by a multi-disciplinary group of social scientists from the University of Leeds, University College London, the University of Liverpool, and the University of Oxford. The projects at Leeds are focused specifically on potential applications of ubiquitous big data to problems in health care, personal mobility, and changing consumer behaviors particularly in relation to environmental attitudes and sustainability. The philosophy of CDRC is to work directly with external partners on problems that are often of mutual interest and typically building from a simple and limited relationship toward long-term data sharing across a wider network of partners. Twenty organizations are now sharing data with a national network of academic partners with the benefit of regulatory mechanisms (including a "research approvals procedure"), which are managed by CDRC.
Some examples can help to indicate the breadth and potential of work now in progress. In studies of health care, our group has been using a combination of individual-based models, hazard estimation, and dynamic transition rules to assess future health care needs. A relatively fine spatial resolution allows such models to inform policy-making options in local environments. Here the advent of new data from wearable devices is potentially transformative in allowing us to understand the effects of modifiable risk factors in relation to diet, lifestyle, exercise, and behavior. Where transport is concerned, again, social media platforms and journey planning apps are starting to provide us with real-time intelligence about where people are, how fast and in what directions. Smart tickets from a bus or train operator can add extra precision in connecting travelers to a specific mode and route. What all of these possibilities require, however, is some element of data integration. A subject who moves relatively short distances over an extended period of jumps and pauses might be assumed to be shopping if this activity takes place in a retail center. A train ride to a university district is more likely to be associated with an educational purpose than one to a city center business district. In the longer term analysis of infrastructure systems, the thinking can again be extended to start "what if?" evaluations of the impact of long-term strategic decisions, ranging from a new bus route or redesigned intersection to a major investment, such as HS2 or a new runway at Heathrow. Similar considerations apply to other forms of infrastructure including energy networks, broadband and communications, water supply, waste disposal, and housing. Ironically, many of the most valuable sources for studies of this type are held by government, which is also the principal decision-maker, but cannot exploit the holdings of organizations such as Her Majesty's Revenue and Customs (HMRC) or the Student Loan Company without contravening the terms under which these data were collected in the first place—a difficulty which the current Digital Economy Bill seeks to address.
In all of these cases, the integration of data is a key consideration. For most social science problems, which are relevant to either policy or more theoretical academic studies, it is unlikely to be the case that all the answers may be found in a single novel data source, but rather that a combination of such sources are required, and through their synthesis the great leaps are now starting to look possible [9]. For example, tackling obesity could involve the accretion of data in relation to genetic characteristics; demographics such as age, gender, and ethnicity; environmental variables like green space; personal attributes e.g., height, weight, and body mass; and activity variables in relation to both lifestyle and exercise on the one hand and diet and nutrition on the other. In the work described here we are typically exploiting "models" (as representations of "theories") which have been constructed and refined over extended periods of time (e.g., microsimulation, spatial interaction models, agents, discrete choice, input-output models, and many more). To be sure some interesting and valuable new methods are starting to gain purchase, e.g., machine learning approaches to the parametrization of models. However any suggestion that some new box of magic tricks labeled big data analytics that sits easily on top of massive new datasets can radically and instantly transform our long-term understanding of society is naïve and dangerous. The excitement about big data for serious social scientists is because it introduces a pipeline to help us refine methods and theories about processes that we have long known to be significant but simply had no adequate handle on before.
A reasonable corollary of this line of thinking is that the variety of big social data is a much more important feature than pure volume. The velocity of new social data is probably more important than sheer scale as well. It is now possible to monitor the impacts of a road traffic accident on congestion in approximately real time, and to do similar things in relation to the spread of an epidemic or the effects of a horse meat scandal on the sale of supermarket ready meals. This provides genuinely transformative possibilities for those with the courage to accept the challenges posed. Essentially we now have the opportunity to build, test, evaluate, refine, and often discard our models against an evidence base that is unfolding continually around us. The bad news for modelers who are willing to engage with this class of problems is that there will be no hiding place for poorly conceived work, but the fruits of success will surely be great. Again new techniques such as data assimilation might be able to help us here.
The quality of big data has been challenged by many, and perhaps proffered by some as an excuse not to engage. Again, variety can be a source of some consolation here. If one source is heavily skewed toward a particular activity or sub-group, then maybe this can be compensated by another source, which has different characteristics. Alternatively it may be possible simply to cross-validate one or more sources of data which are used within the model against one or more data sources of data which are external to it [10]. And here too if one of the cross-validation data sets is drawn from a real-time future which is by definition uncertain then so much the better.
Finally, the broader implications of our earlier remarks about ownership of data are sufficiently important to merit amplification. The privacy and confidentiality of personal data is a great concern to both the individuals concerned and the data owners (and which of these potentially opposing parties has the ultimate legal and moral rights over the data is also a contested question, albeit beyond the scope of this paper). For example, linking health outcomes from a patient dataset with longitudinal consumer datasets on expenditure and diet could lead to huge social benefits in understanding the long-term consequences of lifestyle and lifestyle change. But in the current socio-political and cultural climate the threats to privacy seem to outweigh the benefits. Those who believe in a brighter future with big data need to do more to promote the benefits—the need to demonstrate genuine examples of achievement.
References
[1] Anderson, C. The end of theory: The data deluge makes the scientific method obsolete. Wired 16, 7 (2008).
[2] Savage, M. and Burrows, R. The coming crisis of empirical sociology. Sociology 41, 5 (2007), 885–899.
[3] Goodchild, M. Citizens as sensors: the world of volunteered geography. GeoJournal, 69, 4 (2007), 211-221.
[4] Bell, G., Hey, T., and Szalay, A. Beyond the data deluge. Science 323, 5919 (2009), 1297–1298.
[5] Hakaly, M. and Weber, P. OpenStreetMap: User-Generated Street Maps. IEEE Pervasive Computing 7, 4 (2008).
[6] Procter, R., Crump, J., Karstedt, S., Voss, A., and Cantijoch, M. Reading the riots: What were the police doing on twitter. Policing and Society 23, 4 (2013) 413-436.
[7] Ranco, G., Aleksovski, A., Caldarelli, G., Grcar, M., and Mozetic, I. The effects of Twitter sentiment on stock price returns. PLoS ONE 10, 9, (2015), e138441.
[8] Clark, S., Birkin, M., and Heppenstall, A. Sub regional estimates of morbidities in the English elderly population. Health & Place (2014).
[9] Connelly, R., Playford, C., Gayle, V., and Dibben, C. The role of administrative data in the big data revolution in social science research. Social Science Research 59 (2016), 1-12.
[10] Lovelace, R., Birkin, M., Clarke, M., and Cross, P. From big noise to big data: Towards the verification of large datasets for understanding regional retail flows. Geographical Analysis 48, 1 (2015).
Author
Mark Birkin, Ph.D. is Professor of Spatial Analysis and Policy and Director of the Consumer Data Research Centre (CDRC) in the School of Geography at the University of Leeds in the U.K. His major interests are in simulating social and demographic change within cities and regions, and in understanding the impact of these changes on the need for services like housing, roads and hospitals, using techniques of microsimulation, agent-based modeling and GIS. He is co-editor of the journal Applied Spatial Analysis and Policy, a member of the editorial board of GeoInformatics and GeoStatistics, and on the program committee for the European Social Simulation Association and GeoComputation.
©2018 ACM $15.00
Permission to make digital or hard copies of all or part of this work for personal or classroom use is granted without fee provided that copies are not made or distributed for profit or commercial advantage and that copies bear this notice and the full citation on the first page. To copy otherwise, to republish, to post on servers or to redistribute to lists, requires prior specific permission and/or a fee.
The Digital Library is published by the Association for Computing Machinery. Copyright © 2018 ACM, Inc.
COMMENTS